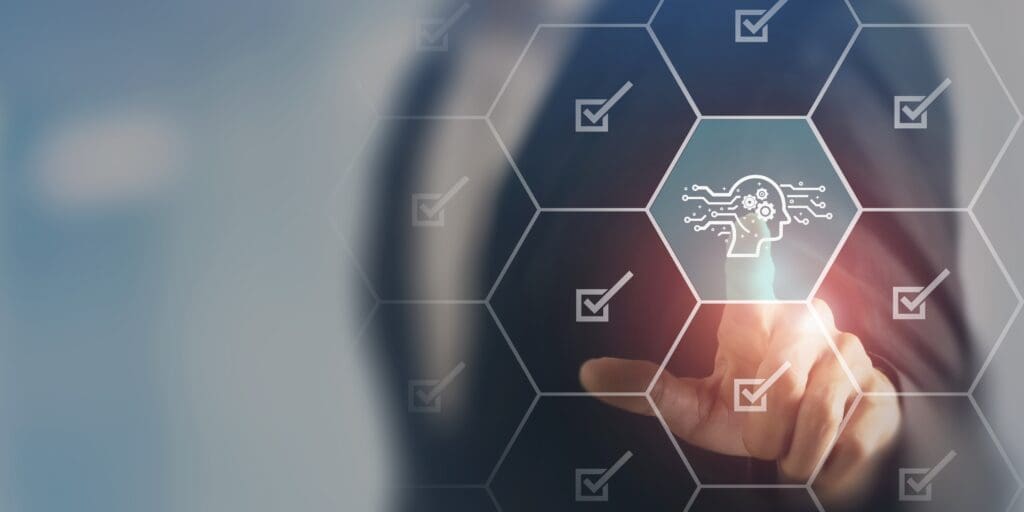
The problem with being a ‘smarter’ MSP is that it may often require investment. For those who don’t have a rainy day fund set aside, it would mean dipping into the revenue to stay competitive and maintain margin, which can be challenging.
As such, finding new ways to save money, such as through automation, can be very refreshing for an MSP. Far too many MSPs still need to make the most of automation. Sure, certain things, such as hardware drivers and software updates, may be automated using management software, and billing is automated via software, but what else can get automated?
Being proactive is a step in the right direction
First, MSPs should look to turn hardware updates from reactive to proactive. This preserves time while also maintaining the performance that customers are looking for.
When being proactive, the MSP should be using data analysis to predict when it is likely that any specific piece of equipment (e.g., server, storage, network router) is likely to fail. Replacing the equipment before it fails will have less impact on the overall platform and greater customer satisfaction. Workloads dependent on that equipment can be reallocated using automation to other parts of the network, maintaining availability.
Likewise, using data analysis helps identify where bottlenecks are beginning to appear. Then, it’s recommended to use automation to offload the workload to another part of the network. At the same time, the bottleneck is removed through the modification or wholesale replacement of hardware as required.
So far, so good. But all of this is simple stuff. How can automation be harnessed to create real differentiation? It comes down to the ultimate answer to everything: artificial intelligence (AI).
But keep reading. I am not one to push the AI story past all modicum of sense. Indeed, much of what I advocate can be seen as being a little more than advanced rules-based engines. However, I would argue that this is the state of AI now – away from the clever generative AI of the likes of ChatGPT, Bard, and so on, we are still in the land of those who can provide very clever rules-based systems.
Using a rules-based approach provides differentiation
So, the prime area that comes to mind for this type of automation is workload flexibility. Aside from flexing the resources that a workload can provide, MSPs can look at providing differential pricing to customers depending on what they want from their workload at different times. For example, a cyclical workload such as payroll can be effectively placed in a deep sleep, almost in offline mode for parts of the week or month. This frees up resources for the MSP that can be used elsewhere to support other workloads.
An example is a customer service workload. The demands on resources here are less predictable. If marketing produced a killer campaign, it could well be that there is a peaking demand for different resources as the campaign kicks off, tailing off as it moves along. The use of rules-based ‘AI’ can ensure that not only does the workload get extra resources, but it can also be moved to a higher-cost and more responsive part of the platform should the customer be willing to pay for it. Once the peak is over, the workload can automatically move back to a lower-cost environment.
So, how about something similar to real AI? Natural language processing systems have become widely used in customer-facing environments to try and parse out what a customer wants before they are passed on to expensive human resources. AI now starts to provide better language parsing and more natural and nuanced responses to the customer, in many cases avoiding the need for the matter to be handed over to a human at all.
Just be careful: the data fed into the model must be clean; you do not want output with casual biases that could upset the customers. You also need to make sure that the outputs are real and factual. This means that AI-based customer interaction solutions need a lot of upfront work to model, create, and check before they can go live.
The same goes for dealing with prospects. Rather than trying to cover everything on a static website, generative AI now means that there is a possibility that prospects can be directly interacted with on a meaningful basis via generative AI chatbots. The same caveats go for dealing with customers – more so, as the interactions here are far more in the public domain.
Now is the time to look at AI for automation
As time moves on and AI matures, bringing all the information together at both high and granular levels will mean that valuable insights can be built up on what prospects are looking for. It also is what makes a prospect become a customer, what makes a customer remain a customer, what services customers are happy to upgrade to based on what they already have, what their business does, and so on.
Now is the time for MSPs that still need to take a real look at AI for automation. Although much of what is available will not be ‘real’ AI, the advanced rules-based systems will provide a good basis for moving forward. Becoming familiar with what generative AI engines can provide can also lead to highly profitable (or at least cost saving) tactical solutions as we wait for fully-fledged AI systems to come along that can be invested in as strategic solutions.
Photo: 3rdtimeluckystudio / Shutterstock
This post originally appeared on Smarter MSP.